Algorithmic Trading
Indicators and tools
Trading systems
Data analysis
Machine learning
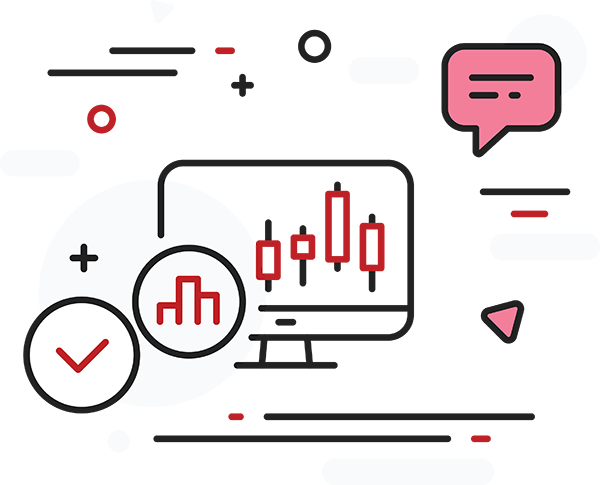
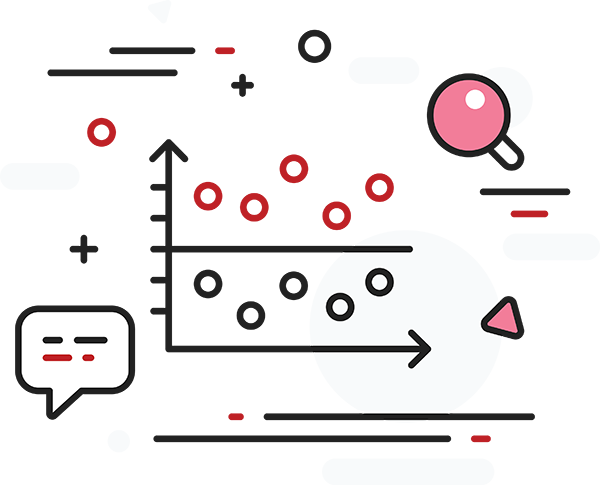
Market Making
As a trading strategy
As a tool for helping exchanges build healthy volume and reputation
For Blockchain projects, creating a liquid market to attract traders and investors
Funds & Investing
CTA strategies
Cryptocurrency HFT strategies
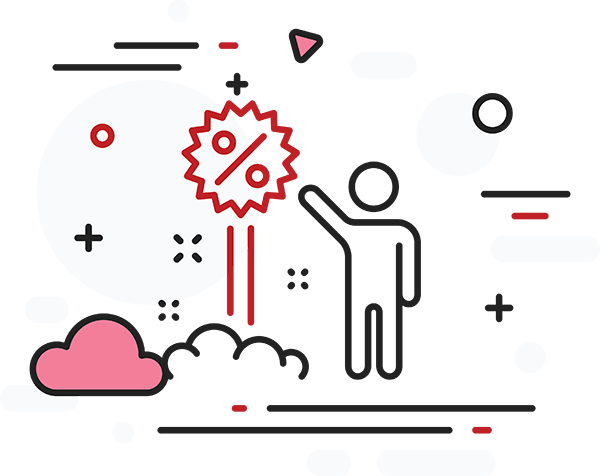
Frequently Asked Questions
What is a trading system?
A trading system is a programmed set of rules that identify entry and exit points for buying and selling (generally) financial products that are priced in a market or exchange.
Why a trading system is preferable over traditional methods?
In the world of finance, investing and trading have been executed over the last century by humans. They analyze the market and make rational or irrational decisions on whether a purchase or sell should be made on a particular instrument. The goal is in most cases to profit from the transaction although there can be other goals as making the market available and liquid for other participants.
This is gradually changing and it is a fact that most successful hedge funds’ decisions are driven today by machines. There are multiple reasons why this could be beneficial but without delving too much into the topic, the main ones are the absence of subjectivity in the decision making, the scientific validation of trading techniques (through backtesting, walk-forward and similar methods) and the scalability that implies automation.
What type of algorithms can be profitable?
The financial markets are a complex discipline, as it is a combination of different subjects: economics, mathematics, psychology… To ask ourselves what combination of rules could be profitable we need to first ask another question: What is the main driver of prices?
It is not easy to find a consensus even between scholars and theoreticians to that question. Some would argue the market is completely random (EMH – Efficient Market Hypothesis) and therefore it is not possible to profit from the markets with better chance than throwing a fair dice. Others would say that they can make money by trading with apparently unscientific or uncorrelated events like moon cycles.
In our experience, markets are definitely non-random, inefficient and therefore there are lots of opportunities. But in order to develop a valid and profitable system we need to apply the scientific method to rational and sound ideas. Otherwise, we run the risk of falling into statistical traps.
What is a statistical trap?
When looking for opportunities in the markets, you would usually test with past data what worked best and what didn’t. This is a key topic and how you use this data will determine the validity of your system (chance of making money).
One of the most common pitfalls is overfitting a system to past data. This can happen when we are looking for a combination of parameters that worked in the past and we test an unlimited combination of inputs without understanding the statistical properties of the sample used and the degrees of freedom of our trading system. Our system might behave very well in the past but when we use new (out-of-sample) data the system does not maintain the accuracy.
Is there any other reason to use automation in trading decisions?
Profiting is not the only reason to work with algorithms in the financial markets. We can put two examples to illustrate how we have worked with clients in similar projects that can be really helpful in their businesses.
– The owner of a financial product might be interested in increasing the liquidity and volume of their ETF or token project. This can improve their visibility, capitalization and cash flow. We are specialized in market making algorithms that provide quotes on both sides of the book to stabilize price and offer liquidity to external investors.
– A hedge fund might have profitable algorithms in their fund, but the volatility is high and this is a problem for attracting investors. An in-depth analysis can explain that holding some volatility instruments or changing the relative weights of their systems can drastically reduce their volatility and increase their risk adjusted returns.